BRISQUE属于无参考图像质量评价的一种方法。无参考就是待评价的图像是唯一的输入,得到一个分数结果。 图像质量是一种比较主观的感受,很难精确的量化。论文就试图用一种方法来量化这个标准。 自然图像(原始图像)像素强度遵循高斯分布,而非自然或失真图像(模糊,噪声,颜色变换,几何变换等)的像素强度不遵循高斯分布。 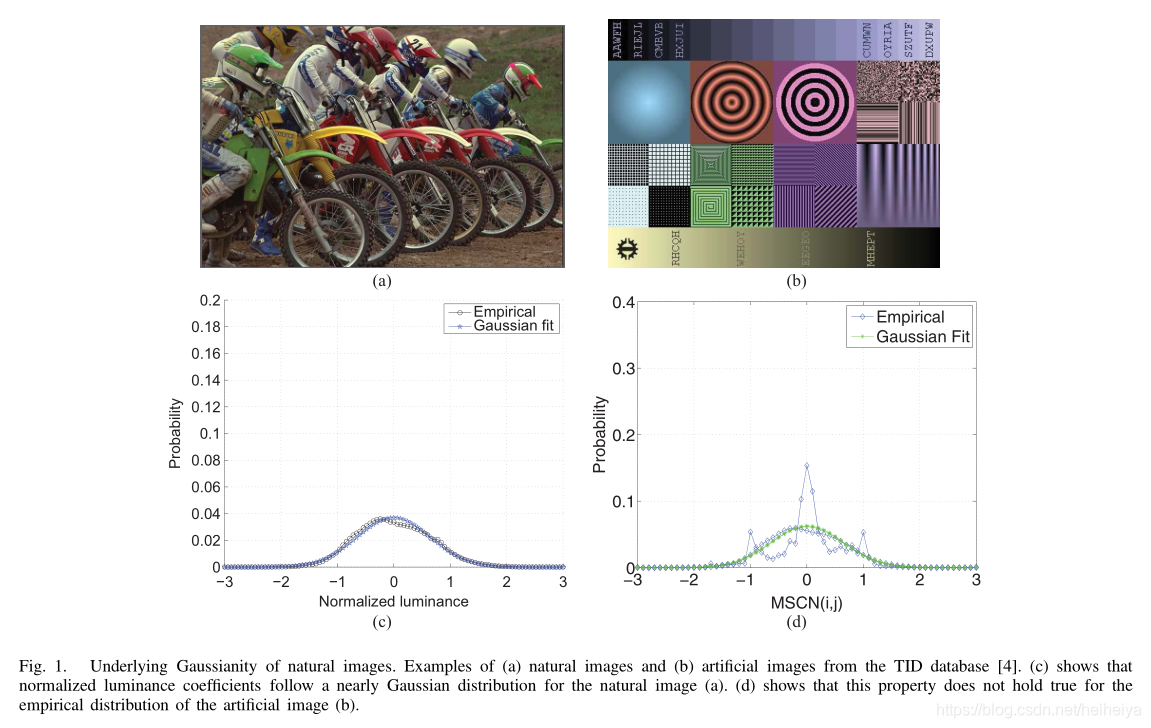 一、MSCN作者使用了Mean Subtracted Contrast Normalized ,MSCN来规范化图像。 
其中, 是空间系数,M,N分别是图像的高和宽。系数C=1是防止除数为0的情况。
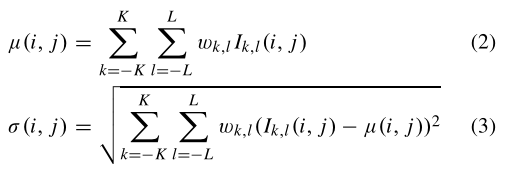
其中, 是二维循环对称高斯权重函数。作者设K = L = 3。 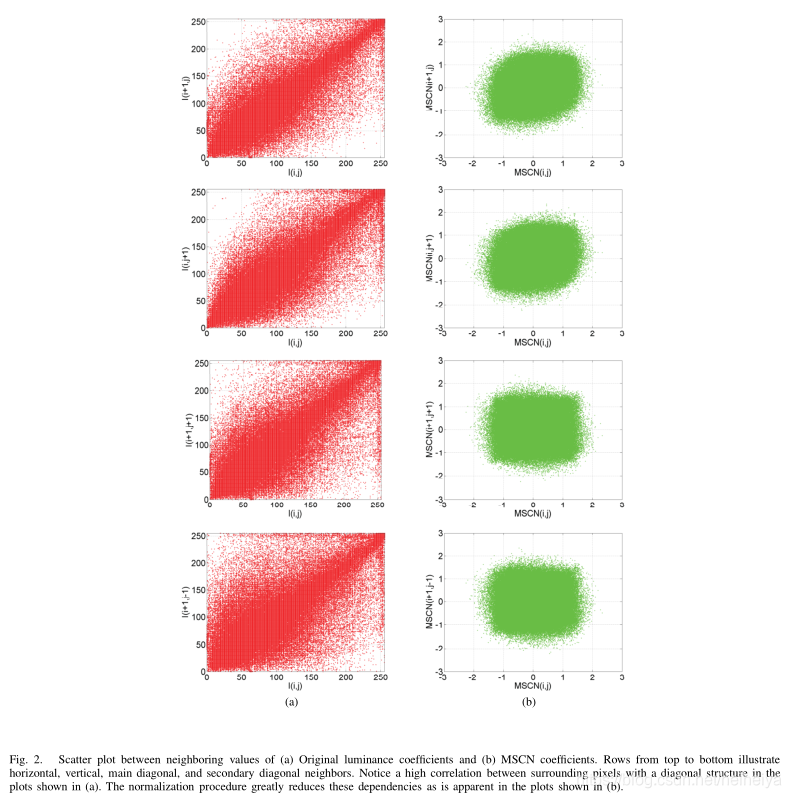
可以看到归一化之后已经明显降低相邻系数之间的相关性。 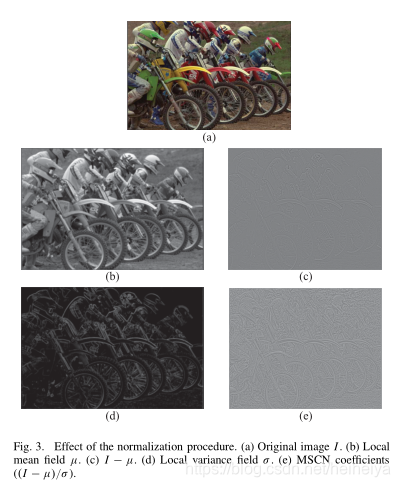
并且对图像纹理的强弱依赖关系也不大。 二、GGD作者假设MSCN系数具有反应图像失真扭曲的统计学特征性质,因此如果能量化这些失真,就使预测这些失真对图像质量的影响成为可能。 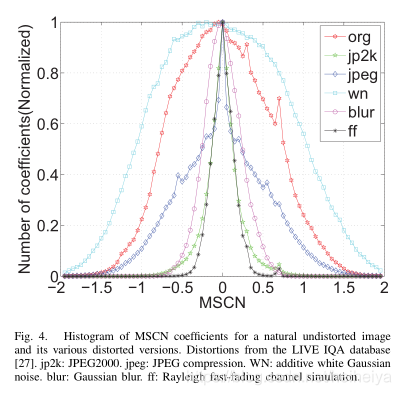
上图展示了不同失真情况下的MSCN系数分布。自然图像服从高斯分布,而失真图像都改变了其统计学特性。 作者发现Generalized Gaussian Distribution,GGD能更好的表示这些失真图像的统计特性。 
其中, 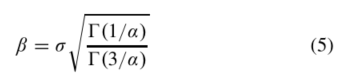

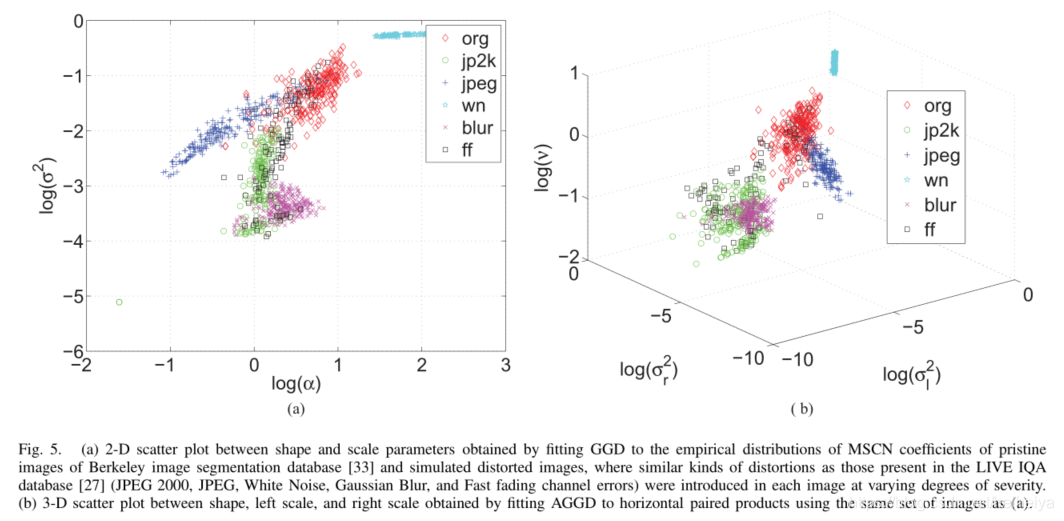 三、AGGD此外,为了捕获相邻像素的关系,作者使用四个方向相邻元素MSCN系数的乘积,分别是水平(H),垂直(V),左 - 对角线(D1),右 - 对角线(D2)。 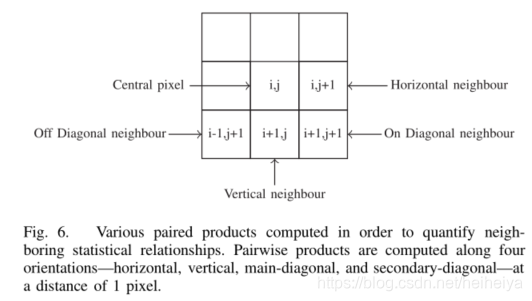
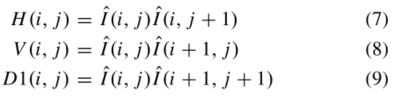

假设MSCN系数是0均值,单位方差,上述乘积在没有失真的情况下服从下面的分布。 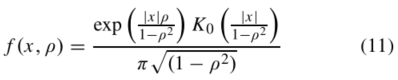
其中,f是非对称概率密度函数,p是相邻系数的相关性系数,K0是修正的第二类贝塞尔函数。 但是这个函数并不能很好的拟合失真图像的相关系数乘积非对称分布。 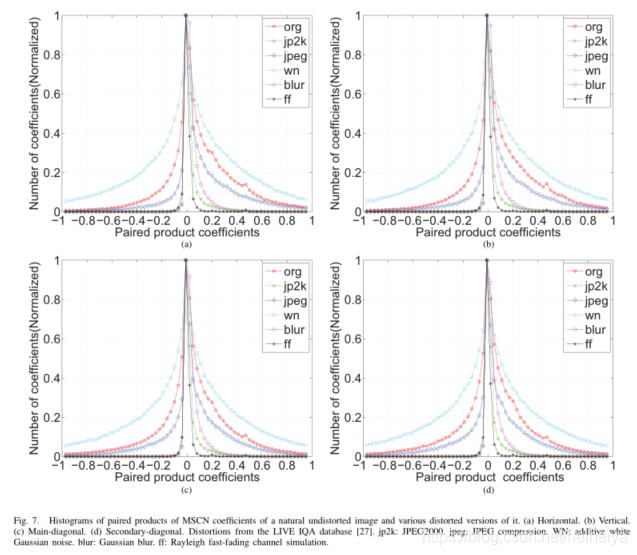
因此作者采用的是Asymmetric Generalized Gaussian Distribution,AGGD。 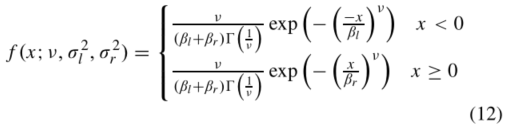
其中, 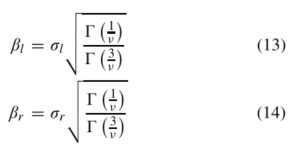
Best AGGD的参数 , 
因此,到这来就可以得到18个features,GGD的2个features,AGGD的4个方向,每个方向4个features。 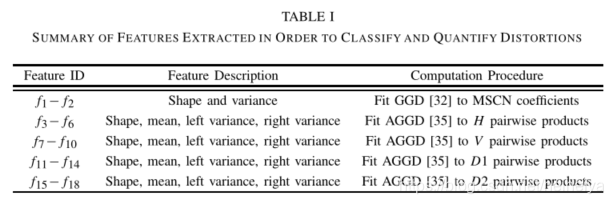
图像通常是多尺度的,尺度也是影响失真的一个因素。有研究证明,将尺度问题考虑进图像质量评价算法比用人的感知表现更好。因此除了在原始的尺度上获取特征,作者还通过低通滤波下采样2倍图像来获取特征(作者实验发现将图像增大2倍并不能提升算法的表现),那么现在在2个尺度上共可以获取36个features。 四、训练作者使用LIBSVM带RBF内核的SVR来训练模型。 数据集由每幅图形抽取的36个features和对应的人为打分送入SVR进行回归训练,使用的数据集是LIVE IQA,划分80%用于训练,20%用于测试。并且进行1000次随机划分训练,最后取中值作为论文的结果。 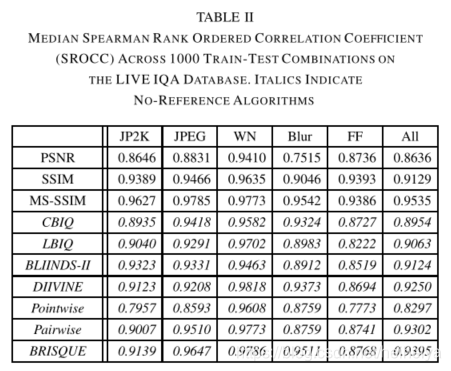
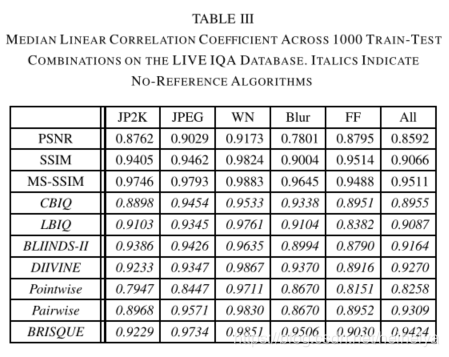
窗口大小对BRISQUE的影响。 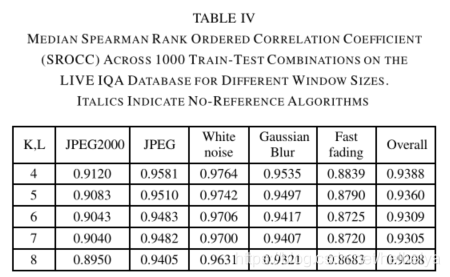
|